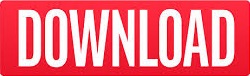
Due to the diverse conditions of rice fields, there are significant variations in the statistical properties of pixels from different images. The performance of supervised segmentation algorithm relies heavily on the quality of the training data set. To the best of our knowledge, no unsupervised learning method has been developed or applied for rice panicle segmentation. Īll above works are based on supervised learning, which requires a substantial number of labeled images for training. In addition to optical images, hyperspectral images have been widely studied for detecting different plant diseases based on machine learning techniques like principle component analysis (PCA) and chi-square kernel support vector machine (chiSVM). More recently, an active learning approach with weak supervision is proposed to reduce the number of labeled training images for panicle detection in cereal crops such as sorghum and wheat. Algorithms mentioned above require a significant amount of labeled training data. Both and use support vector machine (SVM) for detection. Optical images were also used in for wheat ear detection during the wheat heading stage, and in for studying the flowering dynamics of rice plants. CNN-based deep learning algorithms are also used for rice panicle detection in, and for sorghum panicle detection in and. The Panicle-SEG algorithm is trained with a large number of images, from both pot-grown and field plants, to improve its robustness against the diverse conditions of images. In, a rice panicle segmentation algorithm, Panicle-SEG, is developed by using deep learning with convolutional neural network (CNN) and superpixel optimization. The algorithm was developed by using multi-angle images of rice plants, which was rotated on a turntable to obtain images at multiple angles.

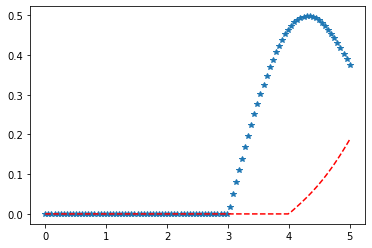
An automated panicle counting algorithm was developed in by using artificial neural network (ANN). Image-based plant phenomics has gained increasing attentions recently. This motivates the development of panicle segmentation algorithms that can operate over images taken under a large variety of conditions. All these factors will affect the accuracy of panicle identification. There are significant variations among images taken under different conditions, such as water reflections, lighting conditions, weather conditions, cluttering backgrounds, panicle rigidness, rice growth phase, rice strains, UAV altitudes, etc. One of the main challenges faced by rice panicle segmentation with optical images is the diverse conditions under which the images are taken. Image-based rice panicle phenotyping relies on accurate panicle segmentation. With the rapid development of unmanned aerial vehicle (UAV) and machine learning, there have been growing interests in high throughput rice field phenotyping by using optical images taken by UAVs over paddy fields. Fast panicle screening can help rice yield prediction, disease detection, nutrition value assessment, precision irrigation and fertilization, etc. The yield of a paddy field is directly related to rice panicles, which are the parts of the plant that carry the grains. More than half of the world’s population depend on rice for their daily calories. Rice is the most consumed staple food on earth. ConclusionsĮxperimental results demonstrate that the proposed method can accurately identify panicle pixels in UAV images taken under diverse conditions. These numbers outperform existing supervised learning approaches. ResultsĪpplying the unsupervised Bayesian learning algorithm on diverse UAV images achieves an average recall, precision and F 1 score of 96.49%, 72.31%, and 82.10%, respectively. The model parameters are iteratively learned by using the Markov chain Monte Carlo (MCMC) method with Gibbs sampling, without the need of labeled training data. The prevalence of each category is characterized by the weights associated with each component in the GMM. Under the Bayesian framework, the distributions of pixel intensities are assumed to follow a multivariate Gaussian mixture model (GMM), with different components in the GMM corresponding to different categories, such as panicle, leaves, or background.
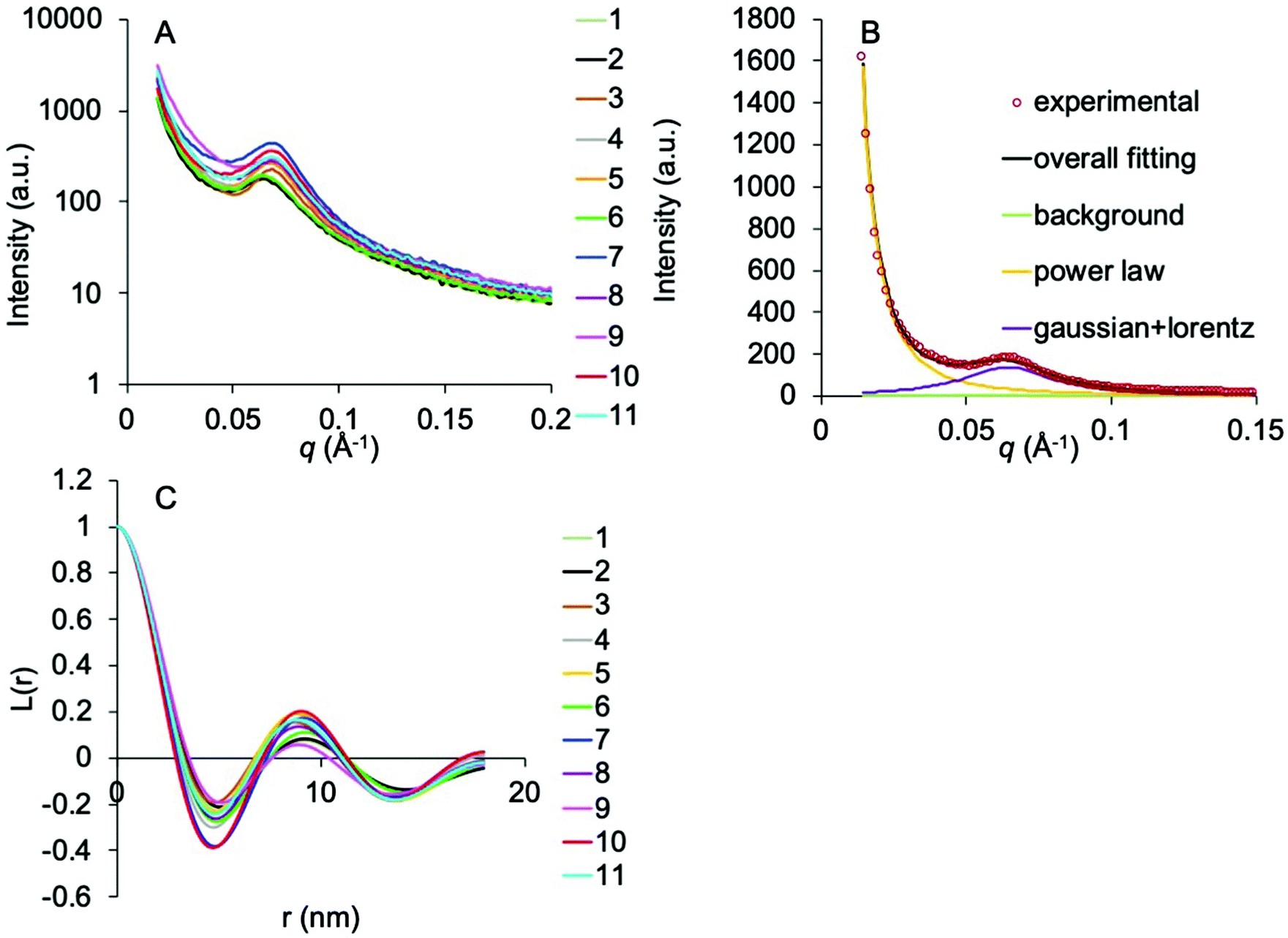
Unlike existing supervised learning methods that require a large amount of labeled training data, the unsupervised learning approach detects panicle pixels in UAV images by analyzing statistical properties of pixels in an image without a training phase. In this paper, an unsupervised Bayesian learning method is proposed to perform rice panicle segmentation with optical images taken by unmanned aerial vehicles (UAV) over paddy fields.
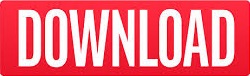